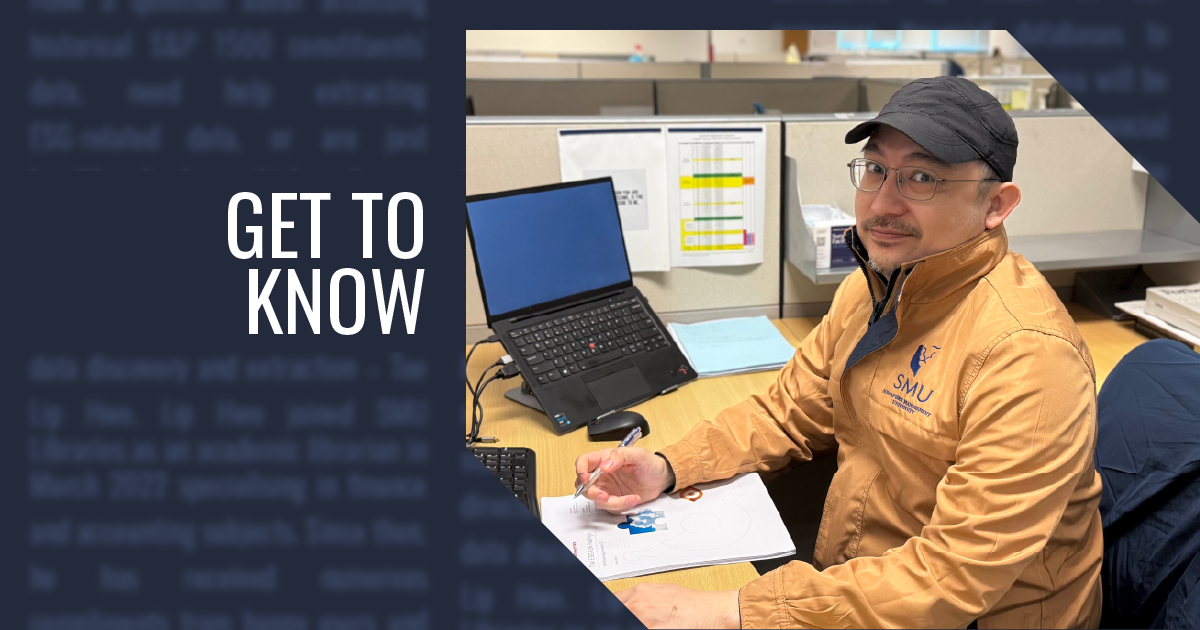
Have a question about accessing historical S&P 1500 constituents' data, need help extracting ESG-related data, or are just bewildered by which of our numerous financial databases to start with? Chances are, you will be directed to our expert in financial data discovery and extraction -- Tee Lip Hwe.
Lip Hwe joined SMU Libraries as an academic librarian in March 2022 specialising in finance and accounting subjects. Since then, he has received numerous compliments from happy users and has quickly become recognised by many faculty and researchers as the "go-to" person in SMU to reach out to for expertise on finance- and accounting-related data.
In this ResearchRadar article, we catch up with him to learn more about his work.
Q: Hello Lip Hwe thank you for taking the time to chat with us. Could you start by telling us a bit about your role as an academic librarian specialising in accounting, finance and business datasets?
I specialize in data discovery and extraction, with a focus on accounting, finance, economic and business data to support academic research. I also work closely with my librarian colleagues to facilitate the onboarding of financial datasets and databases to meet the needs of researchers.
I also conduct workshops, both in-class (on request for finance and accounting modules) as well as bite-sized workshops. Some workshops I have done in the past include "Learning Bloomberg the Fun Way", "Navigating the Data Maze in WRDS: Practical Techniques for Dataset Matching through Identifiers", "Data Extraction from WRDS with Python Programming".
Q: What are some common challenges you see researchers face with financial data? What are some of the issues you face trying to support finance research?
Researchers who ask me for help vary in terms of different levels of understanding in finance and/or skills in extraction and may need help with different things. Typically, though I help them with narrowing down to promising sources that might include the specific data set that might include or be closest to what they are looking for.
Leaving that aside, even the most skilled researchers are often faced with issues and struggle to evaluate the data quality and consistency of a dataset available (e.g. missing or incomplete values; low data granularity) and I work together with the researcher and the data vendor to help determine this.
Of course, often the issue is with data access. Some of the most established datasets have been mined so much that it is hard to find a good use for them, while the newer, "hotter" datasets (e.g. ESG related) are not yet subscribed by SMU and we have to spend time carefully evaluating and trialing to see if they are worth acquiring.
It is extremely hard to predict in advance which datasets will be useful for the SMU community, as researchers themself often find it hard to predict what datasets they might use in the near future.
Also, often, it's not as simple as just buying a dataset, one needs to carefully check for deal-breakers like extraction constraints and data restrictions especially niche or historical datasets imposed by data vendors.
Q: What's one recent success story you can share?
Recently, I was given a fairly challenging question relating to the retrieval of large-scale trading volume data by specific attributes or dimensions to support faculty research.
It took me quite a lot of effort to figure this out, and as per usual I had to spend time understanding the researcher's exact need, understand the data source's data structure and figure out the best way to query and extract the data. I felt a great sense of satisfaction once I figured out how to solve this question!
Q: Where do you see the future of finance research support headed?
Like many areas of research, it is clear that accounting, finance and business related research will be heavily impacted by AI.
Take something as simple as sentiment analysis and textual analysis in accounting research, this was done in the 2000s by counting word frequencies using specific dictionary-based methods (e.g. Loughran-McDonald dictionary) and was then followed by topic modelling methods like LDA in the 2010s. Today, we are using transformer-based type models like FINBERT for various NLP tasks. Similarly, data extraction, NER tasks, will all become more powerful and easier to do.
As such it is important as a librarian supporting finance and accounting research to try to upskill and gain expertise in these areas.
Q: Is there anything exciting that you are working on now that you can share?
Yes, it is my honor to be attending and presenting for the first time a conference - the upcoming International Association for Social Science Information Service and Technology (or IASSIST) 2025 conference in Bristol, UK in June, where I will advocate for a deeper understanding of datasets to uphold data integrity in support of data discovery, extraction, and research.
I am looking forward to share my experiences and network with fellow data librarians and researchers.
Q: Finally, how can readers get in touch with you if they need support or want to learn more?
The best way is via my SMU email at lhtee@smu.edu.sg, but I'm also happy to connect on LinkedIn. My office is at the Li Ka Shing Library, and I'd be pleased to render my support, no matter how big or small.