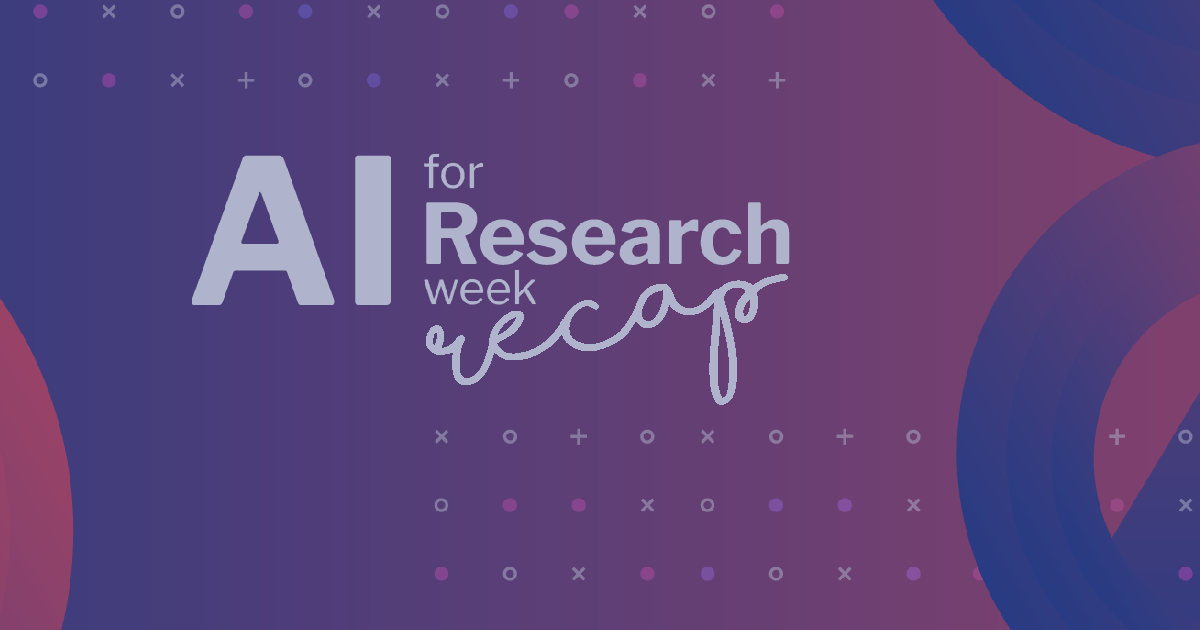
By Aaron Tay, Head, Data Services
We successfully held the AI for Research week from 28th May to 30 May 2024.
What’s the theme? |
Harnessing the power of AI to support your research pursuits. |
---|---|
Why are we doing this event and for what purpose? |
The year 2023 was marked with rapid growth in AI that enables new ways to do research! This has resulted in the emergence of various AI tools for supporting research. This event is aiming to bring together experts, researchers, and enthusiasts to explore and discuss how they can use AI to do academic research and publishing more efficiently and effectively. |
Who was the target audience? |
Postgraduates, Faculty, Researchers, Academics, including librarians in SMU and Singapore. |
What’s the format? Is it a free event? |
|
Throughout the three days, the online sessions had a good turnout, with over 200 participants from 36 countries. Approximately 90 participants attended the in-person workshops, which were only open to the SMU community.
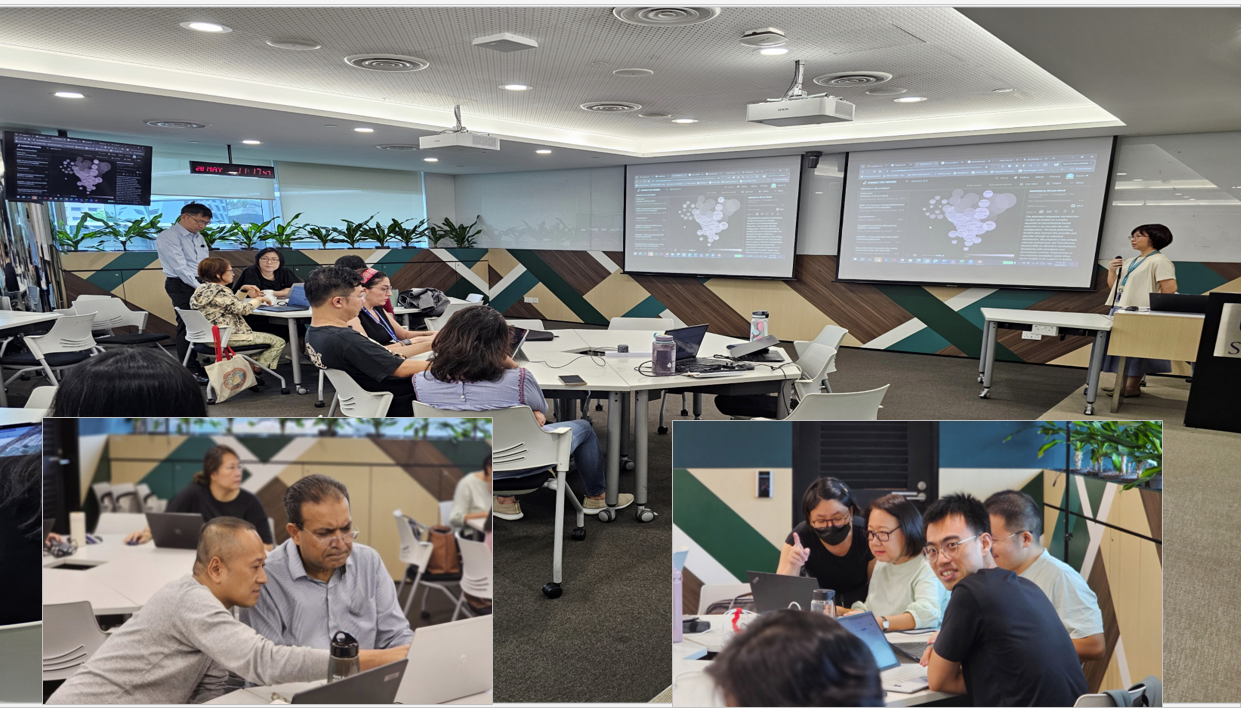
Some selected highlights
There is not enough space for this post to cover all the interesting points and information shared by all the speakers but I will highlight selected points from two talks to give you a taste.
Marc Astbury of Jenni AI provided an interesting talk about how Jenni.ai came about. He talked about how the product could be designed for two different groups of people.
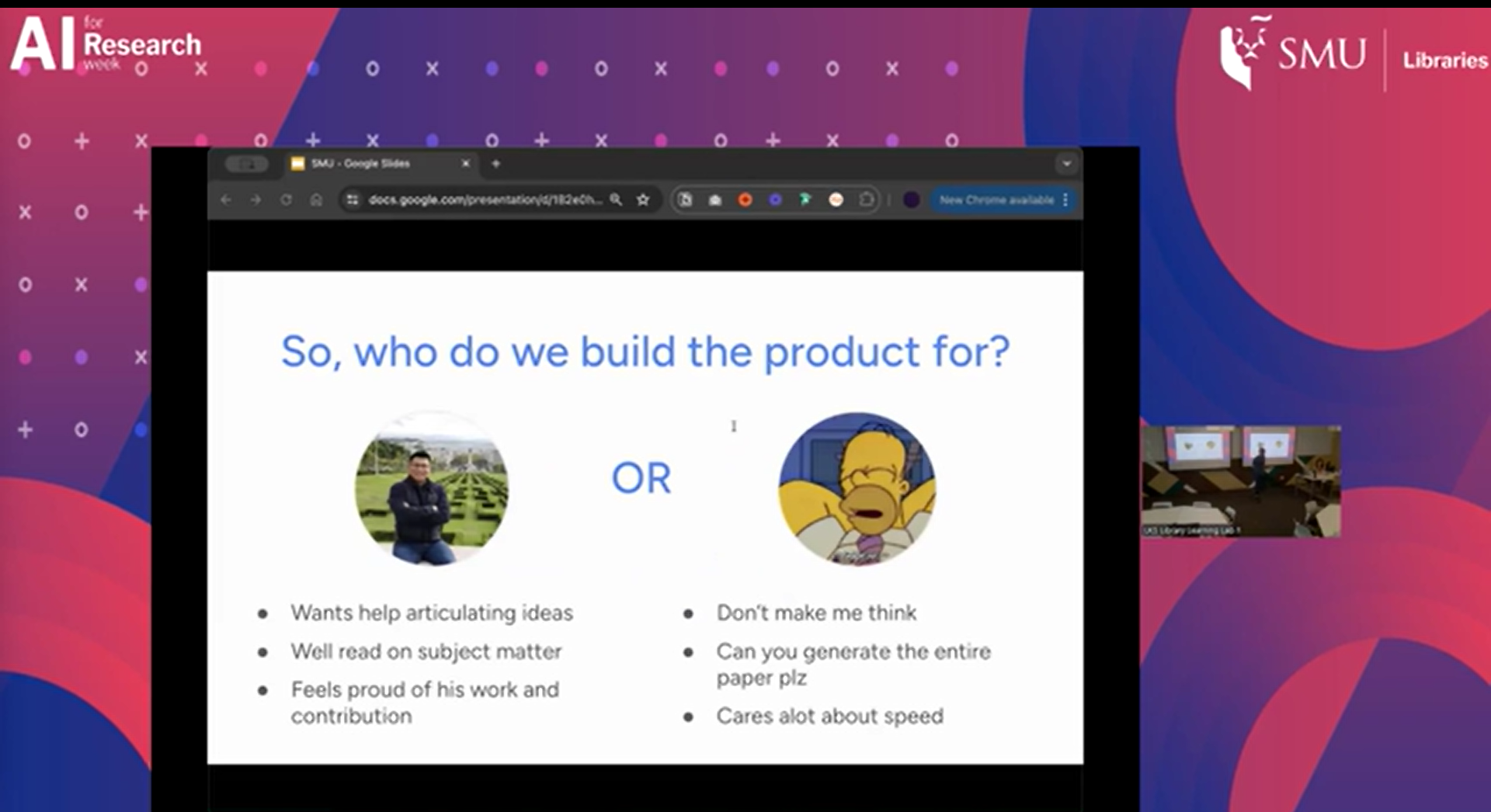
Thinking about these two kinds of users, creates a model or lens through which we can see AI tools as being more of an “AI copilot” vs “AI agent” with the former requiring more human input (human in the loop) and the latter less.
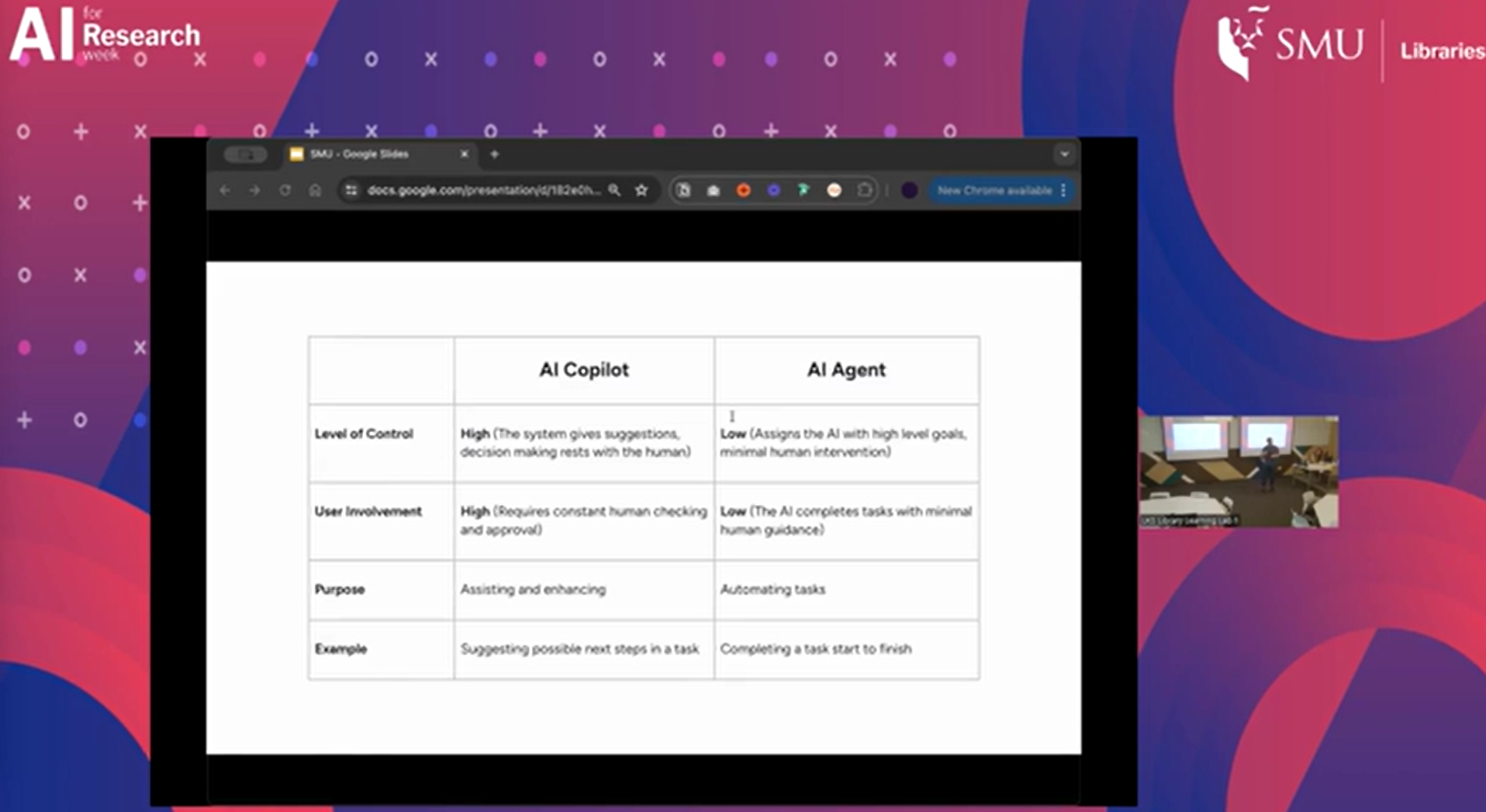
Lucy Lu Wang, Assistant Professor, Information School, University of Washington; Visiting Research Scientist, Allen Institute for AI (AI2) provided a technical yet accessible talk with points and results drawn from her research findings (many of which were presented at top NLP conferences).
For example, she showed the now familiar example of ChatGPT generating statements that hallucinated citations. In particular, she highlighted an "insidious” example where it cited a paper that exists but misrepresented what the paper was about.
She suggested that part of the reason why this occurs is that many large language models are trained on automated metrics that are not accurate.
To unpack this, for many NLP (natural language tasks), such as summarization and Q&A tasks, it is tricky to assess how well the system has done. For example, how do you assess and rate how well a system is able to summaries some text? While you may have a gold standard answer to compare against, there are numerous ways to summarise equally well, so how do you judge?
Worse yet, you need to do this at a large scale, it will be too costly to let humans evaluate thousands if not hundreds of thousands of summarizations. As such, NLP/ML researchers usually resort to automated metrics like ROUGE (various variants), BERTScore hoping that they approximate human judgements and use that as the standard of success.
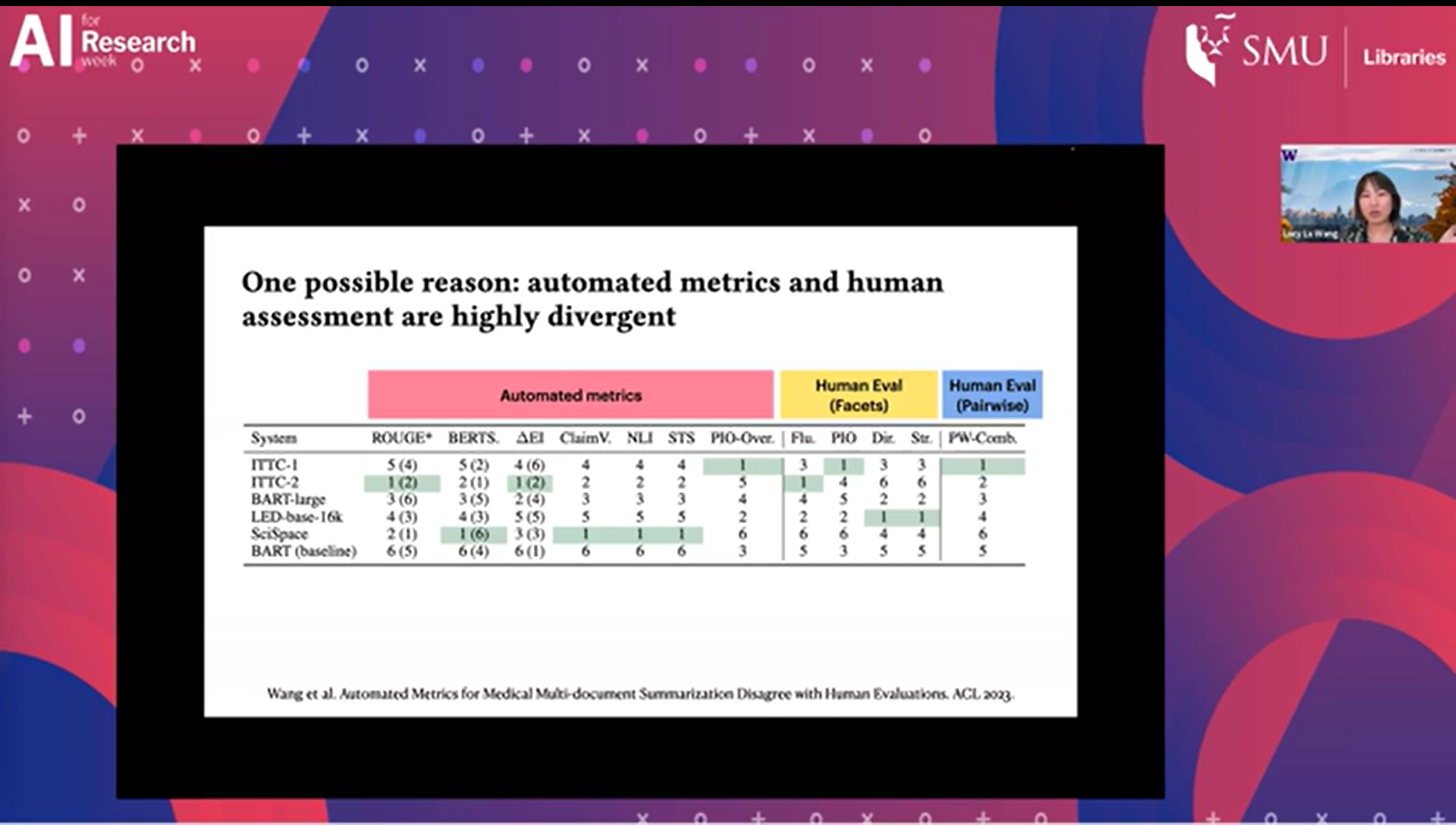
What Prof Lucy found in Automated Metrics for Medical Multi-Document Summarization Disagree with Human Evaluations that the commonly used automated metrics do not correlate with human judgement and in “many cases the system rankings produced by these metrics are anti-correlated with rankings according to human annotators”!
Given that many of these models are trained on, given feedback and selected based on their performance on these automated metrics (that disagree with human judgements), you can see why this might be an issue.
Prof Lucy also showed case her work from a more recent paper - TOPICAL: TOPIC Pages AutomagicaLly.
Her tool which is available a web demo that you can play with is able to generate a topic page with citations extracted from papers (top 10,000) found by PubMed.
It then clusters the paper’s title and abstract using semantic similarity (SPECTER embedding), then sends title & abstract of sampled articles to GPT models for generation of text with prompts. There’s of course a fairly involved prompt pipeline used (see paper for more details).
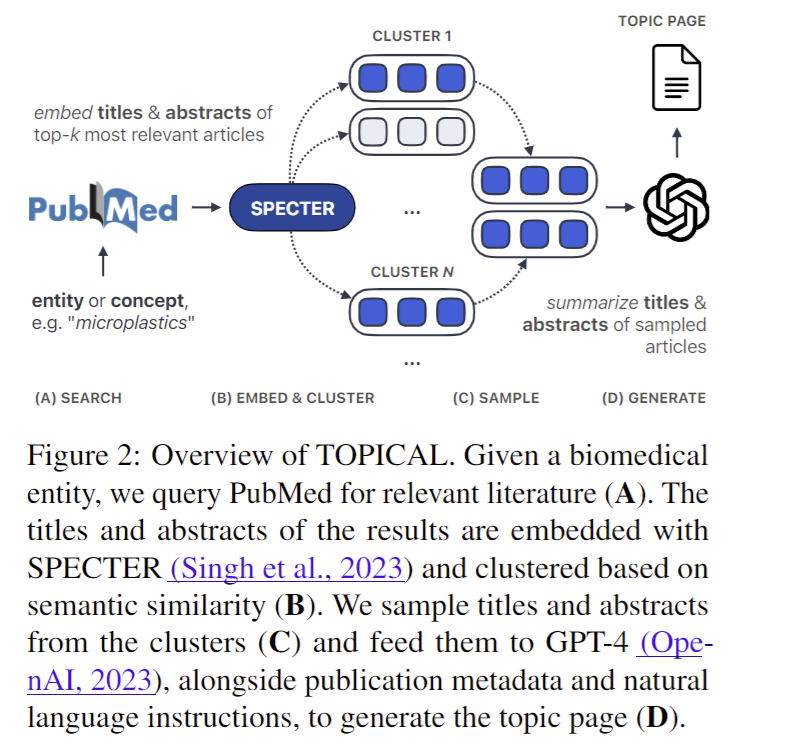
Here’s a sample of results from my play of the tool – TOPICAL.
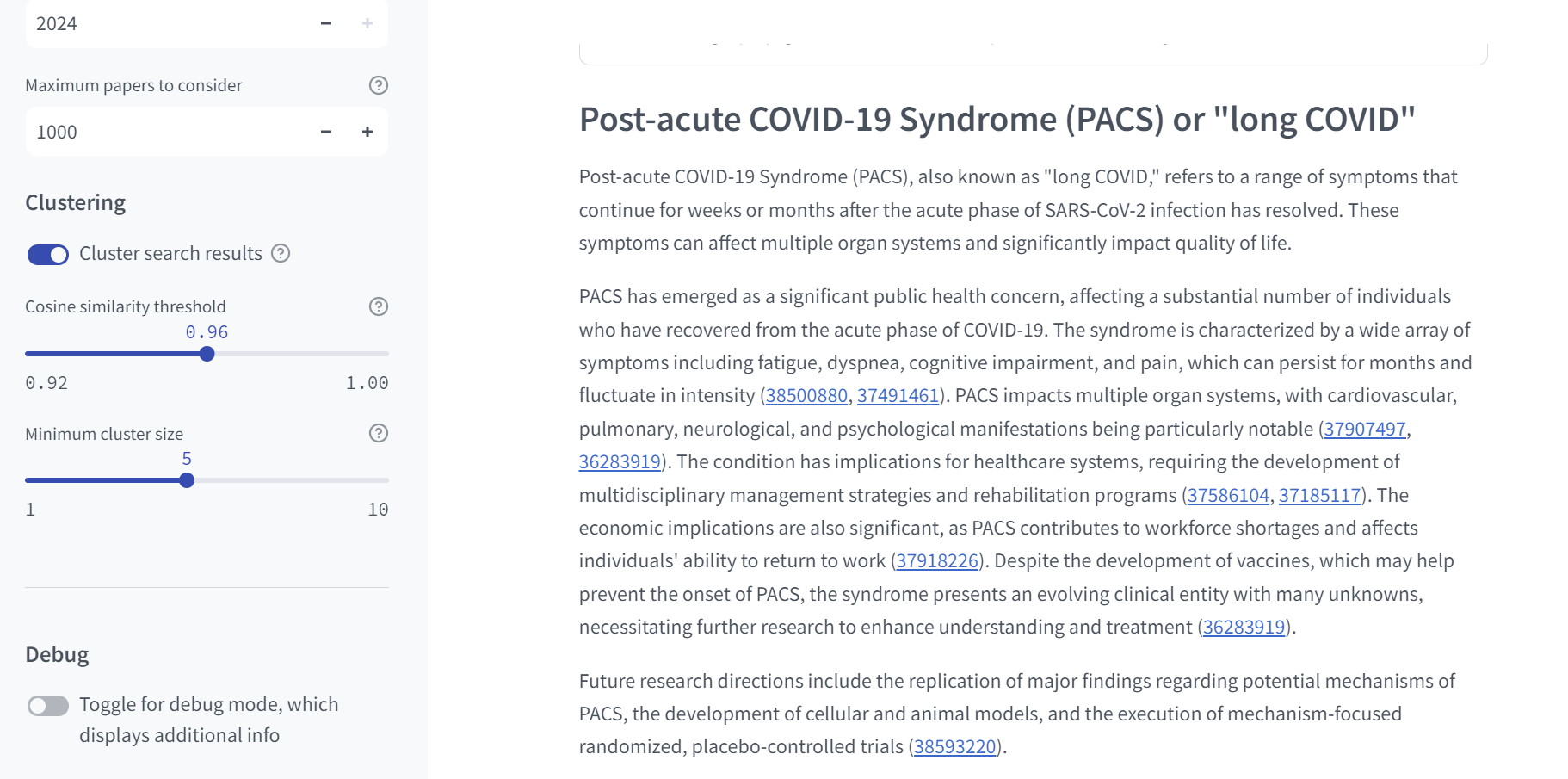
Also, of interest to systematic review librarians, she also showcased a tool, ROBoto2 a tool used to assist in clinical trials risk of bias assessment using Large Language models.
You upload a PDF of the clinical trial and the system tries to find evidence/statements from the papers to address Version 2 of the Cochrane risk-of-bias tool for randomized trials (RoB 2) with a prediction.
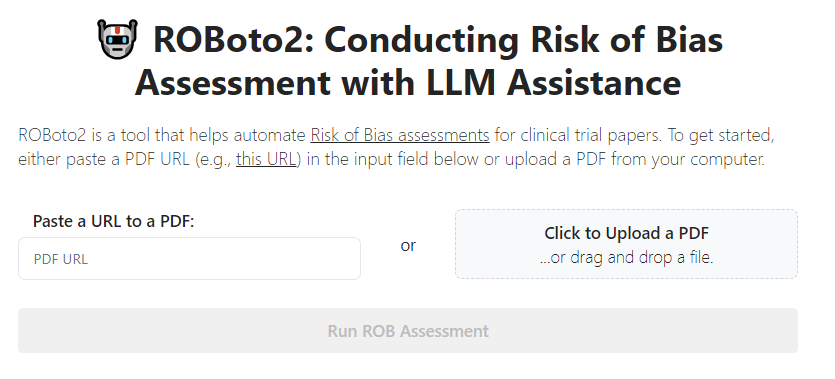
Here’s a part of the output.
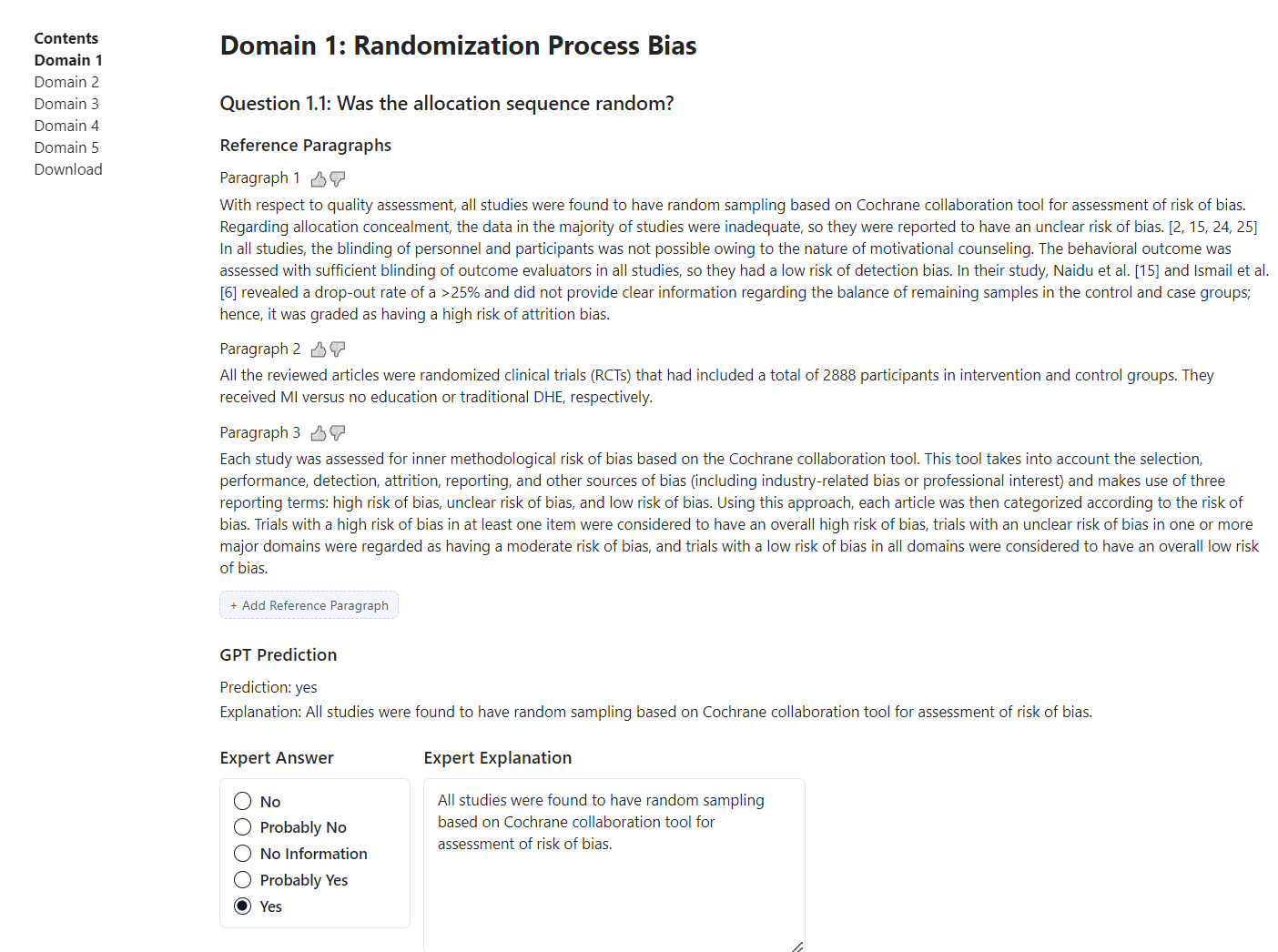
Conclusion
I would like to thank all our speakers, for making the event a success. Thanks also goes out to our librarian colleagues who presented as well as provided logistical support including Danping, Pin Pin, Kooi Ching, Samatha, Bryan, Mui Yen and Francia and most especially Bella who held a dual role as a presenter as well as the project manager. Last but not least, thank you to Professor Archan Misra and University Librarian Shameem Nilofar for their support in opening and closing the event.
AI for Research Week: Presentations and Discussions Recordings